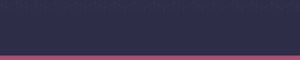
AI in Banking: Building Compliant and Safe Enterprise AI at Scale
Watch this webinar, hosted by Finextra, to explore the importance of aligning AI to banking use cases, data management, and governance. This webinar will discuss:
- How important are open source models in overcoming the challenges banks face regarding data security and compliance when adopting AI?
- In what ways does generative AI bring business value, and how can it be differentiated from traditional AI?
- With efficiency and governance being key concerns for banks, how can synthetic data help to train models while balancing AI infrastructure with customer value?
- How crucial are new regulations like the EU AI Act in light of the need for proper data and model management?
- What role can Agentic AI or AI Agents play in revolutionising banking operations while ensuring compliance and safety
Despite Open AI’s ChatGPT being launched two years ago and mainstream use of LLMs ensuing, many banks and other organisations remain in the early stages of their use of large language models. They are identifying the controls needed to effectively manage their risk, but they are also using new methods to improve their accuracy in real world scenarios. An example is using Retrieval-Augmented Generation (RAG), which optimises the output of a LLM by referencing an authoritative knowledge base before generating a response.
In turn, RAG enhances large language models by improving their accuracy and reducing the risk of hallucinations. While this is a good start, more needs to be done to understand the potential risks on a case by case basis. The emergence of AI Agents introduces new possibilities for automating complex banking operations, offering potential efficiency gains but also raising further considerations regarding compliance and safety.
Scaling AI initiatives from pilot projects to full-scale implementation remains a significant challenge, as many firms struggle to realize AI's full potential. The next step for banks would not be too different from the gradual uptake of cloud: regulators along with the bank’s risk and compliance function need to better understand the risk of this new technology in the context of how it is being used. A good example is the use of privacy enhancing synthetic data used for training and tuning models. This both reduces the impact of a potential data breach, but can also be used to create more accurate models with representative transactional data.
With Meta’s Llama and Google’s Gemini coming to the fore, it is evident that many of the principles of open source will be applied as these new foundational models are created. However, smaller, fit for purpose models will need to be trained and tuned for specific tasks inside the bank, alongside these general purpose models. This is where it is critical to have the right capabilities to effectively manage and streamline deployment of models across the organisation.
Speakers:
- William Caban - Product Manager, Red Hat
- Dr. Richard L. Harmon - Vice President, Global Head of Financial Services, Red Hat
- Skyler Nesheim - CTO, Dwolla
- Sharon Kimathi - Researcher, Finextra [Moderator]